Make the Data Fit the Desired Result
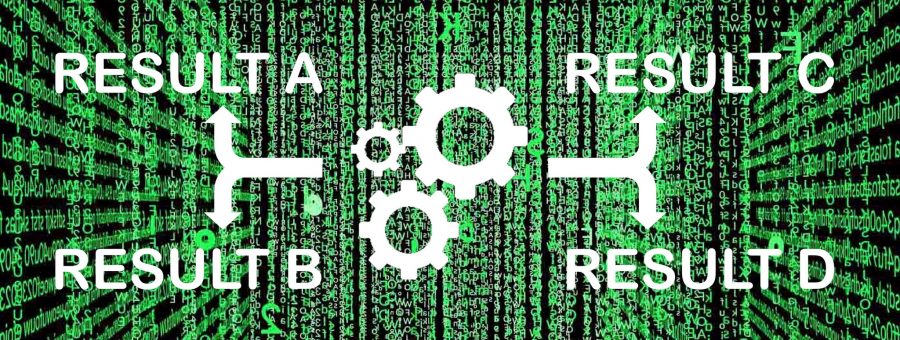
Shôn Ellerton, Aug 2, 2021
Given the same raw data, it is often very easy to spin entirely different stories. And that’s without telling one single lie!
Ever come across those official government results of annual crash fatalities, lives lost due to drink driving, percentage of ethnic minorities incarcerated, or how many lives were lost due to a new disease? Of course, most of us have at one point or another, but how much credence should we place on them?
I am wary of statistical reports showing year-upon-year changes without access to the raw data that supports it. And even if that raw data is available for consumption, how ‘raw’ is that data? Often, the supposedly raw data which supports tables, graphs, and overall statistics fit for public consumption is not raw at all, but already ‘worked’ to fit the overall desired result. The actual raw data that sits behind all the workings is often not available for public consumption.
Why is that?
Data in the public sphere is largely dominated by politics and extensively used as a tool to maintain the interests of those operating within the political system. In the private sphere, corporations keep their raw data under ‘lock and key’ only to then release data to its shareholders and the public at large after ‘processing’ it to best fit a desirable result. ‘Bad’ or undesirable results from raw data is, of course, important to build improvements in the process in which that data is reporting, but it is kept away from ‘prying eyes’. Safety, science and welfare, unfortunately, take a backseat, which is, after all, understandable as human nature tends to look after ‘number one’ over others. This happens at an individual as well as an organisation level.
How is it done?
Making the data fit the desired results is not particularly difficult, especially with large and complex datasets. Many of us who are not data-savvy, assume that the underlying data tables supporting a chart, graph or other visualisation depicting a statistic is true and incontestable. This is often not the case, because to derive those tables, in complex environments, the data may undergo several dozen processes to get there. Moreover, the analysts who request the data often have a ‘figure in mind’ as to what the data should look like, often at the behest of an overarching authority or client.
From school days, we are taught to work out a problem to discover the answer. For example, a teacher might hand out a set of tables depicting the number of rhinos alive and ask the student to give the answer in terms of percentage decline. One works from the raw data to the polished answer. But that does not necessarily happen in complex environments—in fact it seldom does.
For example, to take a previous project I worked on in the past, I was asked to provide data to give to the analysts to report on the percentage of ethnic minorities on a state-wide project. The raw data I worked with came from no less than eight independent sources, many of which had glaring inconsistencies within their own datasets.
My client, the internal analysts, wanted an answer not too dissimilar to previous years’ results. Why? Because as most of us know, if large anomalies or big changes occur, they get questioned. In larger organisations and government, large anomalies and big changes are often problematic because they tend to attract the public eye. Maintaining the status quo and keeping the machinery whirring faultlessly is highly desirable.
In complex data environments, it is often easier to work backwards to fit the desired result, because working forward in the traditional way can result in highly different outcomes depending on the method used. The method of calculating data must be consistent and should be thoroughly documented; however, in my experience, I have observed that this is often not the case. In my example, I was not handed down the method but worked this out from scratch in the traditional forward manner. The answer I provided deviated sufficiently far enough to prod the analysts for me to ‘re-calculate’ again pointing out that a widely differing result will attract undue attention. Bearing in mind the complications of loading and integrating the data from various sources along with the complex procedures which amalgamate the data into more readable datasets, it was not difficult to re-adjust to get to the desired result.
Was I doing anything wrong?
Was I fudging the data?
Was I tampering with it?
No.
Without an agreed method as to how to arrive at the result, I had raw data at my disposal. A lot of it! The data was complex because there were several sources, many of which had contradicting data. Which data is the correct one? Moreover, the attributes and parameters of the datasets had multiple and various meanings depending on who interprets them. I could have come out with a numerous variety of differing results, and, as far as my methodology and workings are concerned, they would all have been correct.
Another interesting topic to touch on is how we interpret the statistics. Many of us are bombarded with statistics daily but how do we interpret them?
Take this example I stumbled on a few years ago when I was researching property in a suburb not far from where I live. Apparently, it had less than half the density of my suburb implying that it was more spaced out with larger properties. In fact, a national real estate website took the bait and interpreted this to be the case, but what the website failed to pick up on was that most of that suburb was taken by a local quarry. The truth, which all the local real estate agencies could have told you, is that the density is near enough identical. What I’m trying to point out is that both answers are correct to a degree. In the former, the answer is correct if one considers if the density was uniform throughout.
There is a point in which deriving the data to suit a particular result becomes demonstrably more difficult or impossible. For example, to suggest that gun crime has increased by ten-fold from last year could be conceivable because the statistic is unsatisfying vague. What does gun crime entail? However, to suggest that premeditated murder using a gun has risen ten-fold in one year without any significant evidence in the underlying data and in a situation in which nothing drastic or unusual has changed (for example, a revolution, a coup, anarchy) would be charged with possibly being erroneous.
I think it’s important to understand that data in complex environments can be interpreted in a vast variety of ways. The more complex the data is, the greater the latitude of presenting the results differently. So, the next time one comes across a statistic, graph or other piece of information which has the potential to alter the interests of an organisation or a political party, remember that it is highly likely that the very same raw data could produce a very different outcome.