The Importance of Understanding How, Why and When Data is Collected
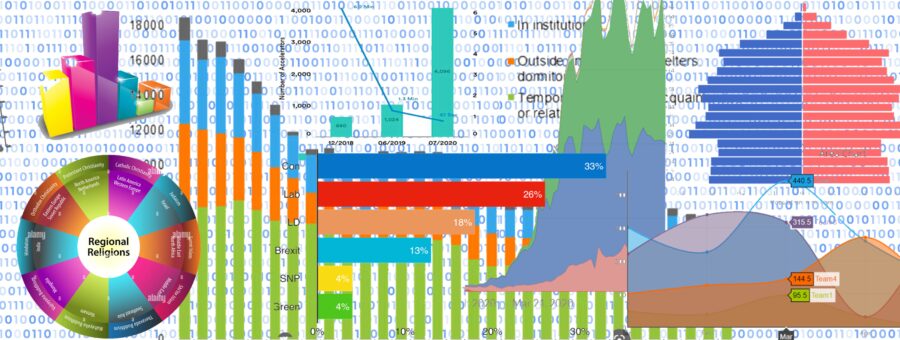
Shôn Ellerton, Feb 16, 2023
Without understanding the when’s, the how’s and the why’s of data, we often find what’s presented to us as useless only to spin a story.
How many people on the planet are having sex at any one time? It was a question raised by the Irish comedian, Dave Allen, during one of his brilliant stand-up routines back in the 90s. He made it out that, if you were an alien looking down on humankind from afar, all you’d see would be a seething mass of wobbling bottoms. He pointed out that, according to statisticians, the average woman has sex two and a half times per week. He then carries on saying how they calculated this, making inferences to secret sex spy agencies with binoculars looking into their neighbour’s windows to take note of any sexual activity which is then reported to some central body of statisticians.
Most of us know how averages work, but Dave Allen takes this to comedic levels when he tries to work out the half bit. Clearly, it would be better to suggest that the average woman has sex five times a fortnight rather than two and a half times a week. Dave Allen hilariously painted a picture of a pervy old man staring through binoculars into the neighbour’s bedroom window reciting his findings as the throes of passion progresses. However, if the action stops short of the pending orgasm, he enthusiastically mutters out, ‘Ha! Must be the half!’ Funny stuff, indeed.
Like many comedians, Dave Allen points out a real dilemma with the world of how we understand data, because if we do not understand the data, we are unable to process this as useful or, indeed, correct information. In my current career as a data architect and database developer, I’ve had plenty of firsthand experiences with this interesting little dilemma. It’s given me an insight how data transforms into information, then on to knowledge, and finally, wisdom. Data is a very valuable commodity, but it is often essentially useless if we don’t know when it came from, how it was collected, and why. Hopefully, we know what the data is. For any grammar pedants out there, I’ll keep with the accepted but unofficial singular form of data because I would never write an agendum!
Those exemplary and learned mathematicians and statisticians armed with complex formulas committed to memory are all too happy to be given heaps of raw data at their disposal to analyse, slice and dice, and spawn a slew of pretty graphs attached with very important messages, some of which are engineered to be tuned to a particular narrative. In the name of, what many misleadingly purport to be science, their findings are submitted to a myriad of peer reviewers to cross-check and verify the results, nit-picking every little finding and that each one they come across has been found by the correct formulas and methodologies. Sometimes, after an enormous amount of energy has been spent on analysing, transforming, calculating, and re-analysing the data, the result could be utter nonsense because the question of how, why and when the data came about may never have been questioned or asked.
In most data transformation projects, the role of the data analyst is to analyse the raw data presented to them. The database developer’s role is to create the data repository to hold and transform the data. This data is then made usable for those who create the reports based on the data. It is a reality that many who fulfil these tasks never question how, why and when the data was collected in the first place. In some situations, questions like these are considered unwelcome, especially in the world of data projects involving confidential and sensitive information. But for most other projects, it is too easy to assume that the data presented to them is the ‘correct’ data and asking such questions is often deemed unnecessary because, surely, someone else must have asked these questions already. It may turn out that nobody has!
For example, a seemingly simple task of calculating the number of railway kilometres owned by a railway infrastructure company may not be so simple at all. Does one count all the little sidings and spurs that are never or hardly used? Are we counting both tracks on a railway corridor or the sum of all tracks irrespective? What about those lines which are disused and those which have been recently built? And in which year? When one looks up how many train track kilometres there are in Germany, for example, we assume that it is taken at time of writing, unless otherwise stated. Many of us don’t stop to consider how this data was collected and the logistics of doing so. Taking another example, a quick search on the Internet reveals that Russia has 85,494 km of total rail lines but makes it clear that this was for 2019 and does not include additional track running down the same line. That may be so, but how was it collected and when? Does the government run a complete and exhaustive run of every line in the country each year? In countries where the rail network is divided into many independent operators like Australia, are we so sure that every operator would make such an exhaustive calculation? Most, I expect, would make deductions and additions on track mileage based on what is being decommissioned or built. However, if omissions and errors are made year on year, the result becomes decidedly more inaccurate.
Within a typical data transformation project, an army of data analysts, data architects, database developers, reporting analysts and project managers may completely skip over the fundamental aspects of understanding how and when the data was derived. And if so, the interpretation of the data could be utterly misconstrued and misleading. Back in 2020, it was reported that Sweden lost something in the order of 5,000 senior citizens in the pandemic within a single month while its Nordic neighbours suffered far less in the number of fatalities. We know, of course, how this happened. Sweden’s mistake with housing older and more vulnerable people in very large old people’s homes, the perfect environment to spread any disease. But within two months, the daily count of deaths had dropped below most other European countries after the problem had been addressed, or, at least, mitigated as best as possible. And this leads to the problem of, not how the data was collected, but when. Sweden’s handling of the pandemic was extremely divisive during 2020 and 2021 and the way of interpreting the data makes a perfect example of twisting the data to suit two entirely different narratives. Those who objected to Sweden’s handling took the total number of deaths regardless of the drastically reduced death count in the ensuing months whereas those who condoned Sweden’s approach in general took solace that, excepting that one terrible month, Sweden might have done the right thing during the rest of the pandemic. The subject of Sweden’s approach to the pandemic has grown very quiet in mainstream circles at time of writing of this article, perhaps indicating some of the biases they once had.
But returning on the fascinating topic of sex, another problem presents itself. The willingness to give accurate data. Thousands of polls take place each year on how people practice sex. Where they did it. How they did it. Do they like other people watching? Do they like having it out in the local park with the ducks waddling nearby? Then you’ve got all the kinky stuff or those practices which may not be viewed by the mainstream as wholesome or even, legitimate sex. Are such questions answered honestly or truthfully? Even with anonymity promised for those taking a sex poll, I guarantee that many may still not answer truthfully.
The lesson I learned with data and how it is interpreted into useful information is to understand the whole lifecycle of how the data was collected, how it was transformed, and ultimately, what the data is going to be used for and why. Can one be overly cautious and sceptical of information portrayed by professional looking graphs and plots written in official looking journals by authors with a string of academic and professional titles? Perhaps. However, in a growing world of ‘armchair experts’ in which one can research just about anything using the Internet, it pays to make best effort in delving deeper by examining not only the presented interpreted information but to probe into entire data ecosystem of how that information came to be. One doesn’t need to have a string of academic qualifications to do this and most importantly, we don’t need to only rely on those who do have them, otherwise known as the logical fallacy of the appeal to authority. If no sources are cited and access to the underlying data is not available, it sometimes turns out that the information given to you is a complete dud and useless serving only to spin a story.